
Wind turbines in clean energy production are like giant pinwheels that convert wind energy into electricity. Some of the strongest and most constant winds are those found offshore, feeding the need for floating wind farms and further research to advance renewable energy technology and methodology.
Dr. Shu Dai, a doctoral graduate from the Department of Ocean Engineering at Texas A&M University, worked alongside Dr. John "Bert" Sweetman, ocean engineering professor, to investigate the methodology of floating offshore wind turbines (FOWT), specifically predicting power, wind speeds and blade fatigue. They theorized that current statistical models of short-term wind processes are inaccurate, leading to poorer maintenance and monitoring of wind turbine performance.
“The new short-term wind speed model we are investigating and developing may change the mainstream method of wind power estimation and blade fatigue analysis,” Dai said.
As he explained, current wind process models, such as those used in TurbSim (a 3D wind-filled simulator developed by the National Renewable Energy Laboratory), assume that the short-term wind process is a Gaussian process. That is, it is a random, or stochastic, process with a normal distribution.
“然而,我们的field wind measurement records suggest that the short-term wind speed process is a non-Gaussian process,” he said. “As a result, the current method of power estimation and blade fatigue analysis is not accurate.”
Dai applied the non-Gaussian process to both field experiments and numerical research in order to prove and remedy these inaccuracies.
Predicting power
He started his research by including ocean environment conditions, such as wind speed, wave height, etc. into the prediction of wind power. To do so, he utilized and processed ocean environment conditions recorded by buoys from the National Oceanic and Atmospheric Administration. He also proposed a new method to predict the mean and standard deviation of power harvested by a FOWT using the statistical parameters of wind processes.
“The FOWT deployed in the ocean is a complicated nonlinear dynamic system,” Dai said. “And the numerical simulation of it costs a lot of computer usage.”
As Dai explained, his technique considers the effects of varying turbulence intensity and higher statistical moments. It also allows the performance of an operating wind turbine to be characterized using a limited number of factors that can be from time-domain simulations or measured field data. Applying this new technique based on random process theory, the ideal power curve of wind turbine can be converted into a practical power curve of different wind conditions. Ultimately, this allows researchers to predict turbine performance in different wind conditions much more quickly.
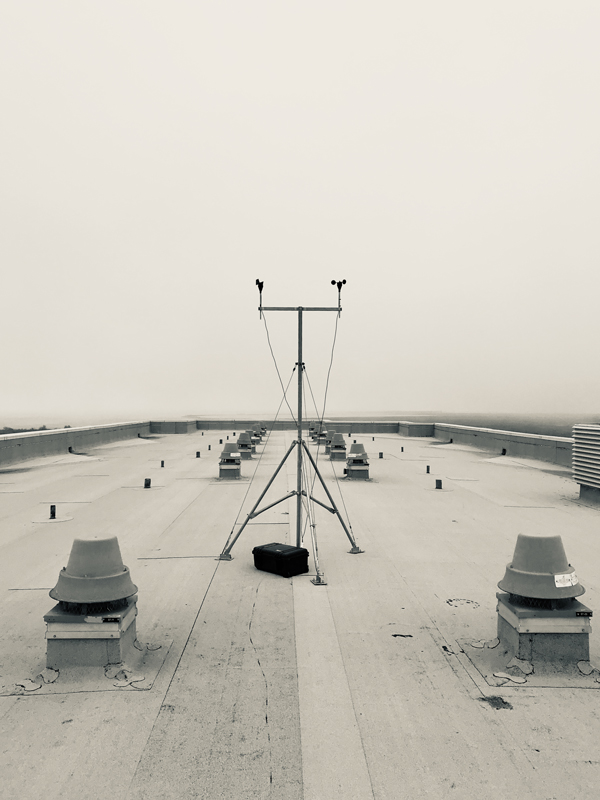
His full findings were presented at the 26th International Ocean and Polar Engineering Conference in a paper titled “Rational Selection of Floater Designs for Offshore Wind Farms Using Power Transfer Functions,” andhas been published by the Institute of Electrical and Electronics Engineers in a paper titled “Transformation of Wind Turbine Power Curves using the Statistics of the Wind Process.”
Recalibrating coastal sea breezes
The second area of Dai’s research takes a small step away from wind turbines; instead, it focuses on creating a new technique for the recalibration of cup anemometers, which are widely used to measure wind speed and direction. Understanding the dynamic motion of cup anemometers, he designed a new recalibration method for the actual wind data captured by such instruments in the field.
In addition to developing the recalibration method, Dai applied this method to field work, conducting two, two-month-long field measurement campaigns on the Texas coast. The coastal breezes were found to be non-Gaussian according to the post-process field records, as he suspected with his work with Sweetman.
His findings will be published in two articles: “A Methodology to Recalibrate Cup Anemometers with Application to Statistical Analysis of Sea Breezes” and Field Measurement Campaigns andIdentification of Sea Breeze and Land Breeze.”
Estimating fatigue
The final aspect of Dai’s doctoral research involved developing a new method of estimating the blade fatigue damage in a non-Gaussian wind field.
Due to the random and turbulent nature of wind, coupled with a saline ocean environment, offshore wind turbines’ structural components undergo various levels of stress that lead to fatigue, wearing down over time and needing maintenance and replacement for the system to function properly.
One aspect of a composite wind turbine imperative for operation is the blade.
“The accurate estimation of blade fatigue analysis helps engineers to choose the best type, material, design, etc. of blades for wind turbines installed at certain locations,” Dai said. “It also tells engineers when to check and replace the parts with potential fatigue damage.”
His new methodology combines several tried-and-true techniques (such as using the OC3-Hywind turbine model to identify fatigue hotspots on blades) with a long-term wind process model (theWeibull distribution) and a short-term wind process model (Gaussian and non-Gaussian) to produce a more accurate and reliable fatigue simulation. Additionally, Dai’s work provides a vital tool for researchers to use to convert the wind field data generated by current models to the non-Gaussian oriented methods that he has developed. It is detailed in his upcoming journal publication titled “Impact of Non-Gaussian Winds on Blade Fatigue Life of Floating Offshore Wind Turbines.”